Hebbian Learning and Negative Feedback Networks: Advanced Information and Applications

Hebbian learning is a form of associative learning that was first proposed by Donald Hebb in 1949. Hebb's theory states that the strength of a connection between two neurons is increased when the two neurons are active at the same time. This theory has been used to explain a wide variety of learning phenomena, including classical conditioning, operant conditioning, and language acquisition.
5 out of 5
Language | : | English |
File size | : | 5786 KB |
Text-to-Speech | : | Enabled |
Print length | : | 401 pages |
Negative feedback networks are a type of neural network that uses negative feedback to regulate their activity. Negative feedback occurs when the output of a network is used to inhibit the network's input. This can be used to stabilize the network's activity and to prevent it from becoming too excited or too inhibited.
Hebbian learning and negative feedback networks have been used in a wide variety of applications, including image processing, speech recognition, and natural language processing. These networks are particularly well-suited for applications that require the ability to learn from experience and to adapt to changing conditions.
Applications of Hebbian Learning and Negative Feedback Networks
Hebbian learning and negative feedback networks have been used in a wide variety of applications, including:
* Image processing: Hebbian learning and negative feedback networks can be used to perform a variety of image processing tasks, such as image enhancement, noise reduction, and object recognition. * Speech recognition: Hebbian learning and negative feedback networks can be used to perform speech recognition tasks, such as speaker identification and speech recognition. * Natural language processing: Hebbian learning and negative feedback networks can be used to perform natural language processing tasks, such as part-of-speech tagging, named entity recognition, and machine translation.
These networks are particularly well-suited for applications that require the ability to learn from experience and to adapt to changing conditions.
Theoretical Foundations of Hebbian Learning and Negative Feedback Networks
The theoretical foundations of Hebbian learning and negative feedback networks are based on the work of Donald Hebb and other early researchers in the field of artificial intelligence. Hebb's theory of Hebbian learning states that the strength of a connection between two neurons is increased when the two neurons are active at the same time. This theory has been supported by a number of experimental studies, and it is now considered to be one of the fundamental principles of learning.
The theory of negative feedback networks is based on the work of Norbert Wiener and other early researchers in the field of cybernetics. Negative feedback occurs when the output of a network is used to inhibit the network's input. This can be used to stabilize the network's activity and to prevent it from becoming too excited or too inhibited.
The combination of Hebbian learning and negative feedback networks has been shown to be a powerful tool for learning and adaptation. These networks are able to learn from experience and to adapt to changing conditions. This makes them well-suited for a variety of applications, including image processing, speech recognition, and natural language processing.
Hebbian learning and negative feedback networks are powerful tools for learning and adaptation. These networks have been used in a wide variety of applications, and they are still being explored today. As the field of artificial intelligence continues to develop, Hebbian learning and negative feedback networks are likely to play an increasingly important role.
5 out of 5
Language | : | English |
File size | : | 5786 KB |
Text-to-Speech | : | Enabled |
Print length | : | 401 pages |
Do you want to contribute by writing guest posts on this blog?
Please contact us and send us a resume of previous articles that you have written.
Book
Novel
Page
Chapter
Text
Story
Genre
Reader
Library
Paperback
E-book
Magazine
Newspaper
Paragraph
Sentence
Bookmark
Shelf
Glossary
Bibliography
Foreword
Preface
Synopsis
Annotation
Footnote
Manuscript
Scroll
Codex
Tome
Bestseller
Classics
Library card
Narrative
Biography
Autobiography
Memoir
Reference
Encyclopedia
Alissa Holder
Brian E Fisher
Michael E Sawyer
Jeff Blackburn
Jeffrey Tucker
Simon Bradley
Robert S Mcelvaine
William Wycherley
Emma Jayne
Lay Hwee Yeo
Ronald L Greer
Sierra Demulder
James Clavell
Matt Morris
Jim Rietmulder
Jorge Galindo Molina
Alina Das
Allan Collins
Larry Frazier
Steven Parissien
Light bulbAdvertise smarter! Our strategic ad space ensures maximum exposure. Reserve your spot today!
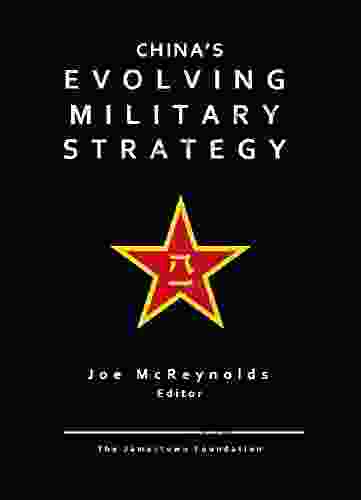

- Justin BellFollow ·15.6k
- Travis FosterFollow ·13.9k
- Henry JamesFollow ·17k
- Dan HendersonFollow ·7.3k
- Dwayne MitchellFollow ·8.6k
- Don ColemanFollow ·8.8k
- Richard SimmonsFollow ·4.2k
- Dan BrownFollow ·17.9k
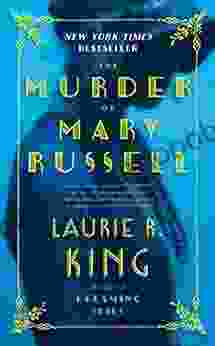

Unravel the Enigmatic Murder of Mary Russell: A...
Prologue: A Grisly Discovery In the...
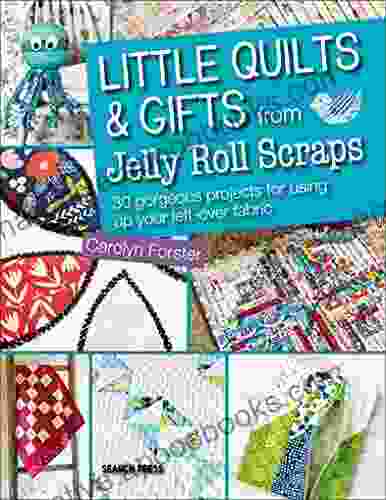

Little Quilts: Gifts from Jelly Roll Scraps
Embrace the Art...
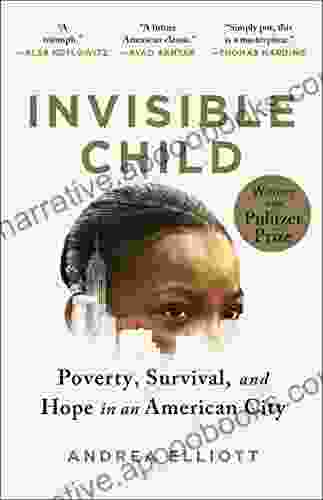

Poverty Survival Hope In An American City: A Pulitzer...
A testament to the resilience of the human...
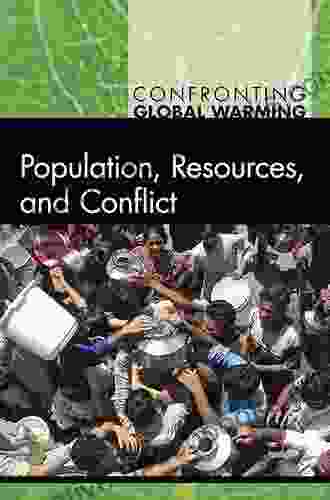

Confronting Global Warming: Population, Resources, and...
Global warming is one of the most pressing...
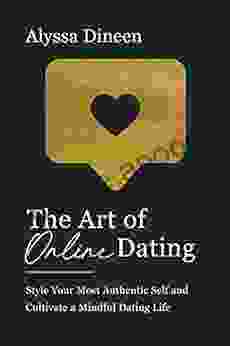

Style Your Most Authentic Self and Cultivate a Mindful...
Unlock Your True...
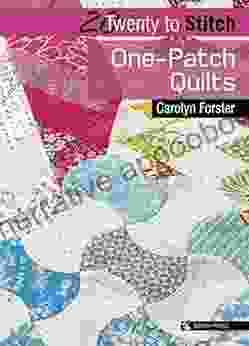

Embark on a Colorful Patchwork Adventure: Discover 20 To...
Step into the captivating world of...
5 out of 5
Language | : | English |
File size | : | 5786 KB |
Text-to-Speech | : | Enabled |
Print length | : | 401 pages |